Generative AI has exploded in popularity across the tech industry, from AI coding copilots to large language model (LLM) APIs powering chatbots. As GenAI products become more widespread, they are also becoming commoditized. Multiple competitors now offer similar AI capabilities, and hyperscalers are leveraging their massive cloud infrastructure to slash prices. A price war is brewing in generative AI, with vendors undercutting each other’s costs and bundling AI features into larger offerings.
In the short term, this battle is driving prices down, a boon for buyers. But the long-term consequences could actually hurt customers, leading to eroded value, consolidation, and less innovation. This post explores the current trend of GenAI pricing cuts (with real examples from the past year), why it’s happening, and why customers may ultimately lose if the race-to-the-bottom continues.
GenAI Becomes a Commodity: The Race to the Bottom
Just a year ago, access to cutting-edge models like GPT-4 was expensive (about $0.06 per 1,000 tokens). Today, prices are plummeting, and many providers offer similar models with only marginal differences. This intense pricing pressure is due to commoditization, with many providers offering similar models, often with only marginal differences in quality or features.
This shift has created intense pricing pressure across the industry, as vendors compete on cost rather than capability. As one analyst noted, there’s “not much to set the various flagship models apart”, which is driving prices lower.
The impact of commoditization is visible in the varying costs per query for different AI models:
- GPT-4: A 500-word response costs around 8.4¢.
- Llama 2: The same 500-word response costs just 0.07¢, a 120x difference.
This price discrepancy highlights why many customers view GenAI as an interchangeable
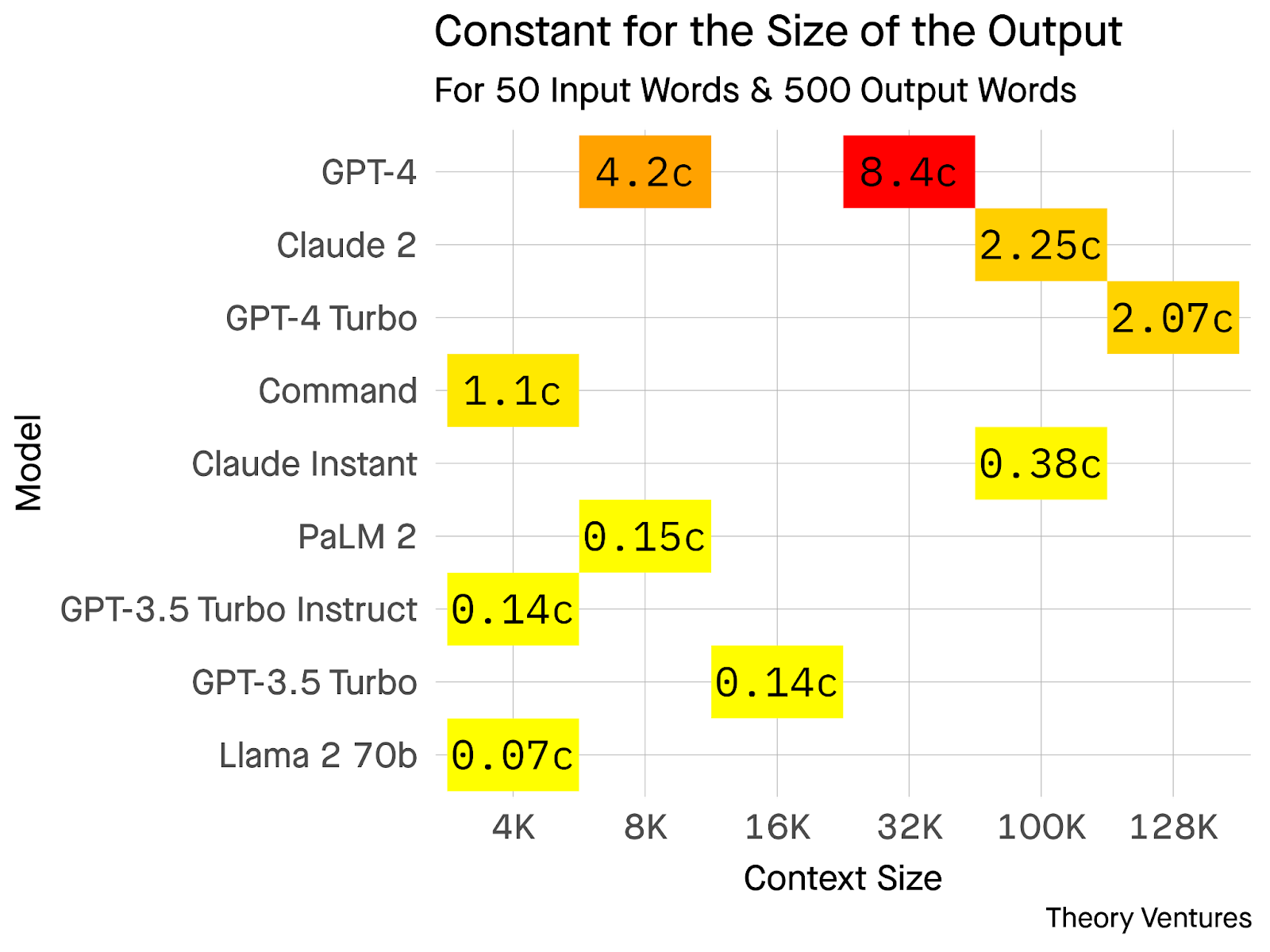
Several factors are accelerating the commoditization of GenAI:
- Open-Source Models: Meta’s release of Llama 2 in July 2023 for free use (commercially as well) has provided a high-quality alternative to proprietary models. Startups and enterprises can now deploy AI models on their own infrastructure, avoiding high API fees.
- Cloud Providers: Major cloud players like AWS, Microsoft Azure, and Google Cloud are running AI workloads at massive scale, reducing their unit costs and enabling bulk discounts. This has further driven down prices.
- Economies of Scale: As cloud providers scale, the cost of running AI models continues to fall. In fact, OpenAI reduced ChatGPT’s running costs by 90% between December 2022 and March 2023, passing the savings directly to API customers. Additionally, data center investments have supported an 86% annual reduction in the cost of inference as of 2024.
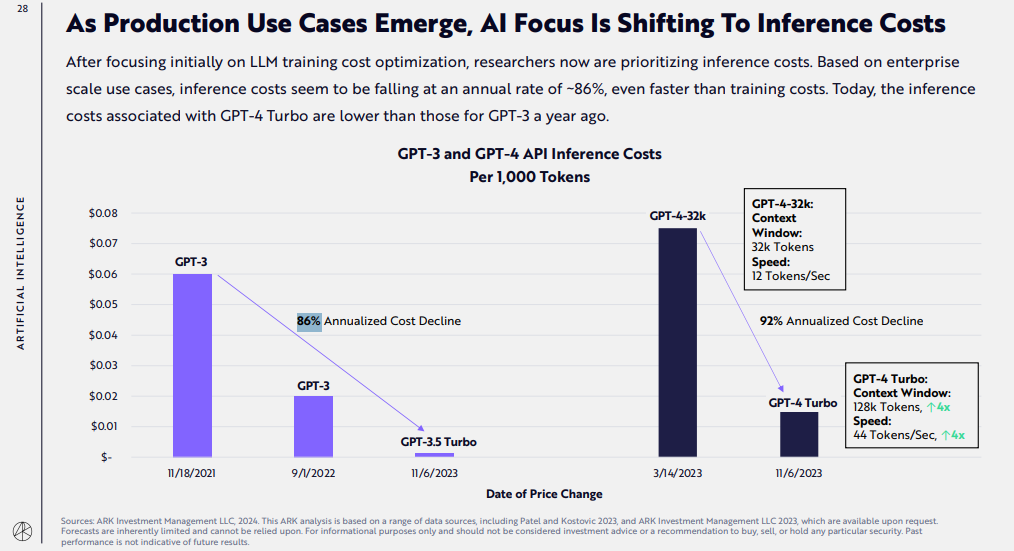
Infrastructure improvements, such as:
- Better chips
- Model compression
- Batch processing of requests
are further allowing providers to continuously lower prices. As these efficiencies grow, so does the ability for vendors to offer more competitive pricing.
A Wave of Price Cuts and Bundling by AI Leaders
Over the last 12 months, we’ve seen dramatic price cuts and bundling strategies from major players:
1. OpenAI’s Drastic API Discounts
In June 2023, OpenAI announced a 75% price reduction for its state-of-the-art text embedding model, and a 25% reduction in the cost of input tokens for the GPT-3.5 Turbo model. This followed the earlier launch of the ChatGPT API in March 2023 at $0.002 per 1K tokens, about 10x cheaper than its previous GPT-3 models.
OpenAI didn’t stop there: at its DevDay in Nov 2023, it unveiled a new “GPT-4 Turbo” model with significantly lower pricing. GPT-4 Turbo’s input tokens came in 3x cheaper than the original GPT-4 (only $0.01 per 1K tokens), and output tokens were 2x cheaper (down to $0.03 per 1K). OpenAI has effectively slashed the cost of AI generation on its platform multiple times in a year, a clear sign of competitive and scale-driven pricing.
2. Google’s Aggressive Moves (And Bundle Strategy)
Google entered the fray by integrating generative AI across its product lines (from Google Workspace “Duet AI” features to its Vertex AI cloud services) and pricing them competitively. In early August 2024, Google reportedly slashed prices on its PaLM/Gemini family models to undercut OpenAI.
Specifically, Google reduced the price of its Gemini 1.5 “Flash” model by 78% for inputs and 71% for outputs. This was in direct response to OpenAI launching a cheaper GPT-4 variant (sometimes referred to as “GPT-4o”). OpenAI had cut GPT-4o’s input cost by 50%, and Google immediately answered by making Gemini 1.5 50% cheaper than that OpenAI model. In effect, Google signaled it will not be undersold on AI API pricing.
Additionally, Google is bundling genAI into its existing offerings, for example, adding AI writing and coding assistance to Google Cloud and Workspace subscriptions (often at no or low incremental cost during previews). This bundling makes the AI “feel” free, upping the pressure on standalone AI service pricing.
3. Anthropic’s Competitive Pricing Tiers
Anthropic launched its Claude 2 model in 2023 as a direct competitor to GPT-4, but with some distinctive perks (like a 100K token context window). It also introduced Claude Instant as a cheaper, faster alternative. By mid-2024, Anthropic’s pricing undercut OpenAI’s in several respects.
For instance, one analysis found Claude’s input tokens to be 95% cheaper than GPT-4’s (and output tokens ~87% cheaper) for comparable tasks. Anthropic even rolled out a flat-rate subscription for heavy users: in April 2025 it announced Claude Max, an enterprise tier offering priority access and higher usage limits for a fixed price, to compete with OpenAI’s ChatGPT Enterprise plans. In short, Anthropic is mixing usage-based pricing with subscription models to lure customers who might balk at OpenAI’s metered fees.
4. Microsoft’s Bundling and Premium Plans
Microsoft’s strategy is to embed GenAI into its dominant software suites, sometimes charging a premium but also adding value that pure-play AI vendors can’t easily match. For example, Microsoft 365 Copilot (announced mid-2023) costs $30/user/month, not cheap, but it’s packaged as an add-on to Office that can leverage your data in Outlook, Excel, etc., delivering more integrated functionality.
At the same time, Microsoft launched Bing Chat Enterprise (an AI chatbot for business web search) at no extra cost for Microsoft 365 E3/E5 customers. Essentially, Microsoft is both charging for AI (in high-value enterprise workflows) and giving it away (in other areas to defend market share).
By offering Bing Chat (powered by GPT-4) “for free” to enterprise users, Microsoft pressures companies like OpenAI that charge per API call. Microsoft knows it can monetize AI indirectly via its cloud and software subscriptions, so it’s willing to subsidize or bundle where convenient. This all contributes to a market expectation that GenAI should be cheap or included, making it hard for independent AI startups to charge significant premiums.
5. Amazon’s Undercutting in AI APIs and Tools
Amazon Web Services joined the fight by introducing its own GenAI platform (Amazon Bedrock) and partnering with model providers like Anthropic and Stability AI. AWS’s play is to offer a menu of models at AWS prices, giving volume discounts to big cloud customers.
A notable tactical move was Amazon CodeWhisperer, the AI coding assistant AWS debuted in 2023. Amazon made CodeWhisperer free for individual developers, directly undercutting the $10/month fee of Microsoft’s GitHub Copilot. The message was clear: AWS is willing to sacrifice short-term revenue to gain users and undercut rivals.
By year’s end, AWS was also offering generous free trials and credits for its GenAI services in an effort to gain market traction against Azure OpenAI and Google Vertex AI. Amazon’s immense cloud scale means it can run these models cheaply, and it’s passing that on in aggressive pricing, because the real goal is to win customers who will stay on AWS in the long run.
These examples barely scratch the surface. Nearly every week there’s news of an AI model price drop or a new “copilot” feature being bundled for free into some platform. Even relatively small players and overseas labs are contributing.
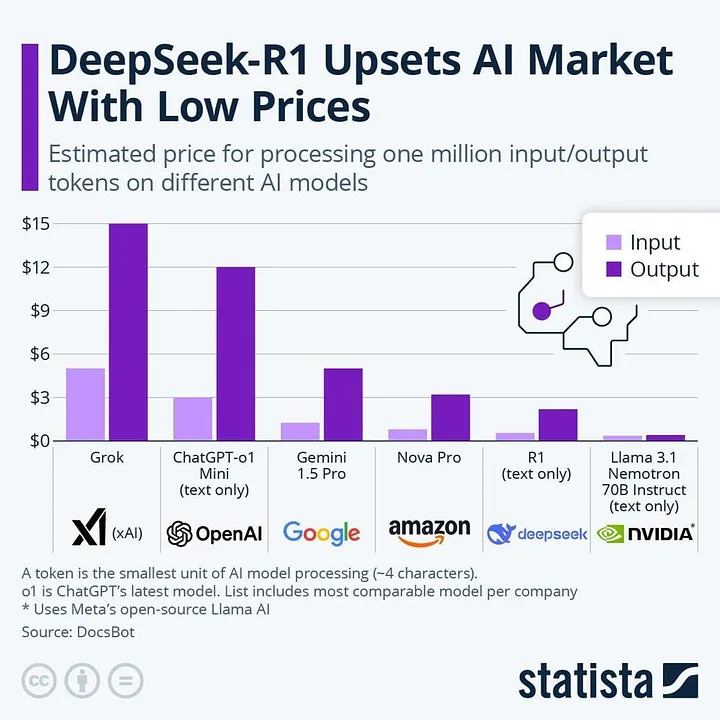
For instance, when a Chinese AI startup called DeepSeek released a model reportedly 20-40x cheaper to run than OpenAI’s GPT series, it sparked so much concern about an impending price war that even AI chip stocks fell on the news. In response, OpenAI and others rushed to emphasize their own cost-efficient offering. The pattern is undeniable: the GenAI market is in a full-on price-cutting sprint, with each player trying to underprice or outvalue the others.
The Immediate Upside: AI Is Cheaper and More Accessible
In the short term, this price war has made AI more accessible, with API prices falling by 65-90%. This makes AI capabilities far more affordable and accessible than ever before, enabling businesses to scale their AI usage exponentially without breaking the bank.
Some concrete upsides of this trend include:
1. Higher ROI and Increased Usage
Companies using GenAI APIs are seeing their margins improve as AI costs shrink. For example, Indian startup InVideo (which heavily uses OpenAI, Google, and Anthropic models) noted that cheaper AI access lets them expand margins, invest more in R&D, hire talent, and improve performance without raising prices. When API expenses drop, you can either lower your own prices or simply enjoy better unit economics. Many SaaS firms have opted to pass savings onto their customers or offer more generous free tiers, spurring greater adoption of AI features.
2. GenAI for Everyone
Lower costs mean AI is no longer just for big budgets. A two-person startup or an academic project can now afford to call GPT-4 or Claude via API, which would have been prohibitively expensive at the old rates. Likewise, enterprises can pilot use-cases with less procurement friction because the spend is minor.
In effect, commoditization is democratizing AI capabilities. We’ve seen a proliferation of AI-powered features in products (from small plugins to large enterprise software) because the input costs are so much lower. This competition has also spurred vendors to offer free trials, credits, or freemium plans for GenAI services that previously cost a lot, reducing the risk for teams to experiment with AI.
3. Better Deals and Bundles
The pricing competition also means buyers can negotiate better deals or get extra value bundled in. If one vendor is charging separately for an AI module, chances are a competitor (or the incumbent if threatened) might throw it in for free to win or retain your business.
We’ve seen this with Microsoft bundling Bing Chat Enterprise at no cost for M365 customers, or Salesforce embedding Einstein GPT features into higher-tier CRM plans. Enterprise buyers have more leverage now, you can push vendors to match the lower prices available elsewhere, or to include AI features as part of the base product. In essence, GenAI is becoming table stakes rather than a pricey add-on, and savvy buyers can capitalize on that.
4. Innovation from New Entrants
In the short term, price wars can spark a burst of innovation as competitors seek any edge to attract customers. Along with cutting costs, vendors are racing to improve quality (e.g. better accuracy, larger context windows) and release new features.
For example, OpenAI’s and Anthropic’s context lengths leapfrogged each other from 4k to 100k tokens in 2023, and open-source models rapidly improved in training efficiency. Much of this is motivated by competition: if everyone’s offering roughly the same price and base capabilities, each player tries to differentiate with something better. The buyer benefits by getting access to steadily improving AI models at a lower price point over time. Even if the core tech is commoditized, the ecosystem around it (fine-tuning tools, AI ops, customizations) is evolving quickly as vendors and startups seek new ways to add value.
In short, the price competition in GenAI is delivering exceptional value to consumers, allowing access to more powerful AI at reduced costs. While some predict further price drops in the coming years, these immediate benefits come with potential long-term trade-offs. Price wars in tech often lead to consolidation, hidden costs, or a reduction in service quality once the competition stabilizes. In the following sections, we'll explore these potential consequences and why, in the long run, winning the price war might hurt customers.
The Long-Term Consequences: Why Customers Could Lose in a Price War
While the allure of rock-bottom prices and widespread AI access is enticing, there are significant risks. Unrestrained price competition can undermine the industry’s health and ultimately harm customers. Here are the major risks and why “race to the bottom” pricing is unsustainable:
1. Eroding Margins and Sustainability
Generative AI isn’t free to provide, the compute and research costs are enormous. If companies keep undercutting each other, someone has to foot the bill. Right now, vendors are essentially trading away margin for market share.
- OpenAI, for example, is reportedly on track to lose ~$5 billion in 2024 due to the high infrastructure costs of serving ChatGPT (despite its API revenue).
- Anthropic, likewise, expects to be $2.7 billion in the red by 2025 if current trends continue.
These kinds of cash burns are not sustainable. Vendors can only subsidize AI usage for so long (even with big VC or parent-company funding) before they must drastically change course, either by raising prices later, cutting costs (which could hurt quality), or exiting the market.
For AI startups, in particular, slashing prices to chase volume is risky if it means negative gross margins. The old days of 80% software gross margins may be “gone” when every GPT-4 query has a tangible cost, now you might only see 60-65% margin, and that’s before any price war discounts. If providers can’t make sustainable profits, customers eventually lose (through bankruptcies, less support, or sudden price hikes when VCs demand returns).
2. Consolidation and Less Choice
Prolonged price wars typically lead to market consolidation. The strongest players (usually those with deep pockets or diversified businesses) can survive a race to zero by subsidizing losses, while smaller competitors get squeezed out.
In GenAI, this could mean that ultimately only a few giants, say, OpenAI (backed by Microsoft), Google, Meta, and Amazon, remain as core model providers. Indeed, the battle for AI model dominance will result in a few winners that capture most of the market’s value. If consolidation happens, customers actually lose leverage: once the upstarts are gone and one or two companies dominate, those remaining providers can start raising prices again or extracting more value, knowing customers have few alternatives. We could also see innovation slow down if new entrants don’t think they can compete with the incumbents’ scale and war chests. A lack of competition is bad for progress.
Today’s plethora of AI model choices might shrink to an oligopoly, as has happened in public cloud infrastructure (the “Big 3” cloud providers) or in internet search and advertising. Fewer options and higher switching costs would hurt customers in the end.
3. Commoditization -> Lack of Differentiation
If every vendor is using the same handful of foundation models (GPT, Claude, etc.) and competing mostly on price, then GenAI capabilities risk becoming a commodity service, undifferentiated and interchangeable.
This has two negative effects for customers:
- First, it becomes harder to find a solution that truly fits your specific needs, because everyone is offering a similar vanilla model with minor tweaks. The incentive to create specialized, high-quality models for niche domains diminishes if those can’t command a price premium.
- Second, products across the market start to feel the same.
For example, if all writing assistants are just reskinned GPT-4 and all code assistants are basically the same model, you aren’t gaining variety by shopping around. True, you might pay very little, but you also get what you pay for, a generic capability with minimal support or unique features. In the long run, customers benefit when companies compete on value and innovation, not just cost. But a brutal price war can kill that motivation to differentiate. We’re already hearing analysts warn that if AI providers don’t find unique value-adds, they’ll be forced into endless pricing pressure to keep users. That’s not healthy for the ecosystem.
4. Reduced Incentive for Innovation
Closely tied to the above, collapsing prices can lead to lower R&D investment and risk-taking over time. If the market conditions imply that no one can charge for advanced capabilities, then making those costly breakthroughs makes less business sense.
Why pour tens of millions into developing the next great model if you’ll be forced to price it on a “cost-plus” basis barely above commodity hardware costs? We could see a scenario where AI advancements plateau because the companies left standing focus on monetizing what they have rather than pushing the envelope (since customers won’t pay more for better).
Smaller AI startups and research labs also might not get funding, as investors worry that any new model will get immediately commoditized by Big Tech. This would be a shame for customers, it could mean slower improvements or innovations that never make it to market. A price war, in essence, taxes innovation, it rewards being slightly cheaper, not being a lot better.
5. Over-Reliance on a Few “AI Utilities”
If current trends continue, generative AI could become dominated by a few hyperscale providers that offer ultra-cheap or free usage as part of their cloud/platform (think Azure OpenAI, Google Cloud AI, AWS Bedrock). Many companies will take the logical route of offloading their AI needs to these utilities rather than training or hosting themselves.
In the short run that’s cost-effective, but it creates a dependency risk. We may wake up in a world where most enterprise software and consumer apps rely on, say, OpenAI or Google’s model behind the scenes. If one of those providers has an outage, a policy change, or decides to tighten pricing once competitors fall away, it could send shockwaves through all the customer apps downstream.
Over-dependence on a few vendors also raises concerns about data governance, compliance, and lock-in. Customers could find it difficult to switch later or to negotiate fair terms when they’ve built entire products around a single model API. Essentially, the fewer the providers, the more power each wields over pricing and service terms. Customers lose autonomy in such a scenario.
6. Service Quality and Support may Suffer
When prices drop precipitously, something often gives, if not immediately, over time. Providers might start to cut corners to reduce their own costs. This could mean less human support (because support is expensive), slower updates for “non-premium” users, or lower SLA commitments on uptime and latency.
We haven’t seen major degradation yet, but anecdotes are emerging of companies noticing rate limits, quality variations, or throttling as providers try to control expenses on discounted services. If you’re only paying peanuts for your AI service, you can’t expect white-glove support when something goes wrong. For enterprises that need reliability, the cheapest option may not truly satisfy that in the long run, and any future introduction of tiered quality (free vs paid tiers) could force a pay-up or suffer choice.
In summary, a prolonged price war could lead to fewer market options, higher risks, and slower innovation, even if services are cheap. Customers benefit when competition drives quality and innovation, not just price. A price spiral that cuts margins will likely result in a few surviving giants, who will need to recoup their losses at customers' expense. As said: “AI at bargain prices, but who’s really paying the bill?” Today’s discounts are often backed by outside funding, but once that runs out, customers will bear the cost.
However, this outcome isn’t inevitable. Both buyers and vendors can influence how the market evolves. In the next section, we’ll explore how enterprise buyers can avoid falling into the low-price trap, and how founders can differentiate through innovation rather than pricing alone.
What Enterprise Buyers Should Do: Think Beyond the Price Tag
For enterprise tech buyers, the GenAI price war might seem like a dream come true, with vendors falling over themselves to offer lower costs. But it’s important to think long-term and not be blinded by short-term savings. Here are some recommendations for buyers evaluating GenAI tools and platforms:
1. Prioritize Value and Fit, Not Just Cost
When comparing GenAI solutions, look at the overall value and outcomes, not just the per-unit price. For example, Model A might be half the price of Model B, but if Model B is more accurate or fine-tuned for your domain, it could drive better results for your business. A cheaper coding assistant that mis-suggests code 20% more often could cost more in developer time than it saves in fees. Consider metrics like accuracy, reliability, security, and integration ease, these differentiate offerings far more than a few cents difference in API pricing.
2. Assess Vendor Viability and Roadmap
A tool being cheap today is no good if the vendor disappears tomorrow or stagnates the product. Especially when considering startups or new entrants with ultra-low pricing, do some due diligence. Are they well-funded or generating sustainable revenue? Or are they in a cash-burning race that might flame out? Prefer partners who have a credible plan for balancing growth with sustainability (not just the lowest bidder).
Also discuss the vendor’s roadmap: are they investing in improvement or just cutting prices to chase users? You want to hitch your wagon to a provider that will be around and innovating in two years, even if it costs a bit more now.
3. Beware of Lock-In via Bundling
Tech giants are bundling GenAI features into broader offerings (cloud credits, SaaS suites) which can be very convenient. By all means, take advantage of a good bundle deal, but keep an eye on portability. If you go all-in on, say, Azure OpenAI Service because it’s bundled with your Azure contract, ensure you have contingency plans or at least exportable data/models to switch later if needed. Avoid architectures that make it impossible to swap out one AI API for another. Maintaining a level of vendor independence is a smart hedge in case pricing or terms change unfavorably down the line.
4. Consider Total Cost of Ownership
The sticker price of the AI service might be low, but what about the total cost to implement and use it effectively? One solution might require a lot more engineering effort to integrate or more prompt tuning by your team, that’s a cost. Another might handle data privacy compliance for you, whereas a cheaper one leaves that burden on your IT department.
Sometimes paying a bit more for a managed, enterprise-grade service saves money when you factor in internal labor, oversight, and risk costs. Calculate ROI holistically. In many cases, a higher-priced (or not heavily discounted) option can still yield better ROI if it delivers superior outcomes or saves you time.
5. Support a Competitive Ecosystem
This is more of a philosophical point, but enterprise buyers collectively have influence on the market. If everyone only ever chooses the absolute cheapest option, we collectively send a signal that price is the only thing that matters, which will fuel the negative cycle described earlier.
By contrast, if buyers show willingness to pay for things like data security, customizability, or performance, vendors will continue to compete on those attributes. When evaluating proposals, don’t automatically eliminate a vendor because they are a bit pricier, engage and let them justify the value. You might even be able to negotiate a bit off the price without driving them to unsustainable levels. The goal is to get a good deal, not to bleed your vendors dry. Long-term partnerships where the vendor can succeed financially tend to result in better service for you, too.
In short: do your homework and play the long game. Leverage the favorable pricing environment, but choose GenAI tools that align with your strategic needs and have staying power. Don’t just chase the lowest token price. In a few years, you’d rather be with a slightly more expensive provider that’s thriving and supporting you, than have to scramble because the “cheapest” option went bust or stopped improving.
What GenAI Founders Should Do: Compete on Value, Not Just Price
As a GenAI-powered SaaS founder, the pressure to compete on price is immense, especially when large players like AWS, Microsoft, and Google can afford to outmatch you. However, focusing solely on price is a dangerous game, you can't win against these giants in the long run. Instead, focus on differentiation, efficiency, and sustainable strategies to stand out.
1. Identify Unique Value Props
Ask yourself, what makes our GenAI product special apart from the underlying model?
- Maybe you specialize in a particular industry (e.g. legal AI, medical AI) and have proprietary data or domain expertise that makes your output better for those users.
- Maybe your UX is deeply integrated into customer workflows (so it’s not just an API call, but a whole solution).
- Or you offer strong guarantees around data privacy, latency, or customization that generic APIs don’t.
Lean into those differentiators and charge for them. Customers will pay more when they perceive real, tailored value that they can’t get from a generic service. Competing on value means you’re not selling just “GPT-4 access,” you’re selling a solution to a problem, of which the model is one component.
2. Don’t Race the Giants to Zero
Be very cautious about pricing your product unrealistically low just to win deals. The cloud giants or well-funded labs can afford to outloss you, they can cross-subsidize AI with other revenue streams (cloud contracts, advertising, enterprise licenses). If you engage in a direct price war with them, you will likely lose unless you have an extraordinary cost advantage.
Instead of trying to undercut everyone, consider value-based pricing: charge according to the value you deliver to customers (perhaps via tiered plans, usage bundles, or outcome-based pricing) rather than purely usage-based commodity pricing. Many successful SaaS companies use a mix of per-seat or tiered pricing plus usage. That way, you aren’t solely valued on a per-API-call cost. You might, for instance, include a certain amount of AI usage in a subscription and charge extra for high volume, but your base fee covers the unique capabilities, support, and integration your product provides.
Avoid the trap of advertising “our AI is 50% cheaper than OpenAI” as your main pitch, that invites a losing battle on a playing field defined by the big guys.
3. Leverage Open-Source and Cost Innovations
To survive and thrive, optimize your own costs aggressively. Use open-source models (like Llama 2, etc.) when feasible to reduce dependency on expensive third-party APIs. Many startups are now fine-tuning open models to achieve ~90% of GPT-4 performance at a fraction of the cost, this can protect your margins and let you pass some savings to customers without bleeding.
Also invest in technical efficiencies: prompt caching, batching of requests, selecting cheaper models for low-stakes tasks, all the tactics OpenAI and Google themselves use. The more you control costs, the more flexibility you have in pricing. You can then sustainably offer competitive pricing and maintain a healthy business. In essence, try to build moats around cost (through IP or infrastructure savvy) and around quality. If you have a genuine cost advantage because of a tech breakthrough, that’s different from just cutting price as a marketing ploy.
4. Focus on Customer Experience and Trust
Another way to differentiate is by providing an exceptional customer experience around the AI. That includes better onboarding, premium support, hands-on fine-tuning help, robust privacy and compliance assurances, etc. Enterprise customers, especially, will often prefer a vendor who offers hand-holding and reliability over a bare-bones cheap service.
If your AI product can be positioned as a trusted partner rather than a commodity API, you can justify a premium. Build features that enterprises care about: user management, audit logs, on-prem deployment options, fine-tune on their data securely, things the general APIs may not offer readily. This steers the conversation away from “how many cents per token?” to “how will this drive outcomes in my business?” where you want it to be.
5. Resist Unrealistic Pricing Pressure
In competitive sales situations, you might face prospects pushing you to match a larger rival’s discount. It’s okay to say no or walk away from deals that would be grossly unprofitable. Not every customer is a good customer if they expect you to set yourself on fire to keep them warm.
Instead, educate your buyers (many will read this post too!) on the value differences beyond price. If you truly can’t win without underpricing to a damaging level, consider whether that is the right segment to target. It might be wiser to refocus on customers who appreciate your value or a niche where you can set the terms.
Hold your pricing confidence when it’s justified, some discounting is normal, but don’t let your young company be dragged into unsustainable arrangements by the market’s noise. A few strong, profitable customer relationships are better than dozens of loss-making ones that drain your runway.
6. Collaborate and Partner Strategically
Finally, realize you don’t have to go it alone against the giants. Consider partnering with larger platforms in a way that leverages their scale rather than competes head-on. For instance, some startups strike distribution deals with cloud providers (offering their solution through the cloud marketplace) to reach customers together, the big cloud provides the cheap infrastructure, the startup provides the specialized layer.
Or partner with consulting firms and integrators who can amplify your reach and help make the case for your product’s differentiated value to clients. By fitting into the ecosystem, you can avoid direct price wars and instead ride the wave of AI adoption. Just be careful in partnerships to maintain ownership of your unique IP and customer relationships so you’re not easily commoditized or replaced.
In short, differentiate or die (financially). The GenAI landscape will reward those who either have the lowest cost at scale (a game for the giants) or who create unique value on top of AI. As a startup, choose the latter. Competing on value doesn’t mean you ignore pricing, you should still be prudent and competitive in what you charge, but it means you’re selling something customers can’t just get from the lowest-cost provider. If you play that game well, you can avoid being a casualty of the price war and even carve out a loyal customer base willing to pay for outcomes and excellence rather than just raw compute.
Conclusion: Navigating the Coming Storm
The GenAI price war is heating up, with offers like free tiers for high-end models, “unlimited” plans, and bundled deals making it harder to justify standalone AI products. While customers enjoy short-term benefits of lower prices, the economics of AI will eventually require someone to pay. We may see a market shakeout, leaving a few dominant platforms with greater pricing power but less incentive to innovate. It’s crucial for customers to remain vigilant to avoid a future with limited options and stagnation.
For buyers, it’s important to capitalize on competition without becoming overly reliant on an unstable market. Support vendors that offer real value, and be mindful of the long-term impact of your decisions, don’t expect the price war to last forever.
For AI product builders, the focus should shift to differentiation and operational efficiency. Competing on price alone against larger players is unsustainable. Instead, prioritize customer outcomes, tailor solutions to specific use cases, and leverage smart technology.
At Monetizely, we believe a successful product strategy goes beyond pricing. Competing based on outcomes and ROI will always be more sustainable than a race to the bottom. While some companies may seek short-term growth through deep discounts, those focusing on differentiation and long-term value will capture lasting benefits.
The message is clear: be strategic. Buyers should ask if their partner will continue to innovate after the price war ends. Founders must create products so valuable and integral to customers that they’ll remain the choice, even when cheaper alternatives emerge. By focusing on innovation rather than short-term price cuts, we can ensure the GenAI revolution delivers enduring value and progress.